AI Mastery for Data Engineers: Advanced Research Techniques to Go Beyond Basics
Equip yourself with high-level research methods to choose the best tools and design scalable architectures.
Exclusive Prizes for Data Enthusiasts—Enter Now!
This issue of Data Gibberish is sponsored by ME!
I’m thrilled to share a chance for you to win some fantastic prizes worth over €370!
Here’s what’s up for grabs:
🎁 5 x 1-Year *Data Gibberish Pro* Memberships
🎁 3 x €20 Amazon Voucher
🎁 1 x 1-Year Subscription to Benjamin Rogojan's newsletter
✨ 𝗕𝗼𝗻𝘂𝘀: Everyone who enters receives a 1-month Pro membership to my newsletter.
Greetings, curious reader,
There's no shortage of articles and tutorials on prompt engineering and basic AI concepts. But as a data engineer, you probably feel like all these resources scratch the surface and are AI-generated themselves.
How do you put AI to work in data engineering? How do you find the right tools, design scalable architectures, and implement practical solutions in a real-world environment?
In this series, you and I are diving deep. We won't cover the basics of prompt engineering or elementary AI concepts — there are plenty of generic resources online.
Instead, I'll focus on what data engineers need to integrate AI effectively. This week, I will show you who to:
Find the best tools for specific tasks.
Learning from how big companies design their data architecture.
Creating your own robust, scalable data architecture.
I have two sets of prompts today:
Basic for everyone
Advanced for Pro Data Gibberish members.
But here's the thing: Basic prompts are good enough. You can do a decent job even without supporting my work.
Wanna see the advanced Library? Check this page.
Last but not least. I am using Perplexity for this week's article. But they do not sponsor this article. They don't even know I exist. There are no affiliate links whatsoever. I genuinely use (and pay for) Perplexity.
I'm very excited. Let's get started!
Reading time: 10 minutes
Research Level #1: Researching and Selecting the Right Tools
Choosing the right tools for data engineering projects is crucial. There are so many tools available, from open-source to proprietary. Each has its strengths and weaknesses. As a data engineer, you need a structured approach to evaluate and select the best option.
Choosing the right tool is a complex process. We can dive deep another time. Now, I will focus on the research using AI. Here's how I do that:
Defining Your Requirements
Start by clarifying your project's requirements. Use the following checklist to make sure you cover all essential aspects:
Data Volume: What's the estimated data volume? Is it in the range of gigabytes, terabytes, or petabytes?
Processing Type: Do you need batch processing, real-time streaming, or both?
Scalability Requirements: Will the tool need to handle spikes in data or scale as the company grows?
Budget: What's the available budget? Can you afford a paid tool, or is open-source essential?
Integration Needs: Does the tool need to fit within an existing tech stack?
Real-World Exercise #1: Use Perplexity. Create a document where you outline the requirements for an upcoming or hypothetical data project. Be as detailed as possible, listing requirements for data volume, latency, budget, and compatibility. This document will serve as the foundation for evaluating tools.
Here's the basic example prompt:
I need you to list 20 data integration tools. Here are my requirements:
- Supporting 10 billion records per day
- Batch workload support
- Built for the cloud, possibly with Kubernetes
- Prioritise free and open-source tools
- Support for Salesforce, NetSuite, S3 as sources and Snowflake as target
And here's a part of the answer for the more complex prompt:
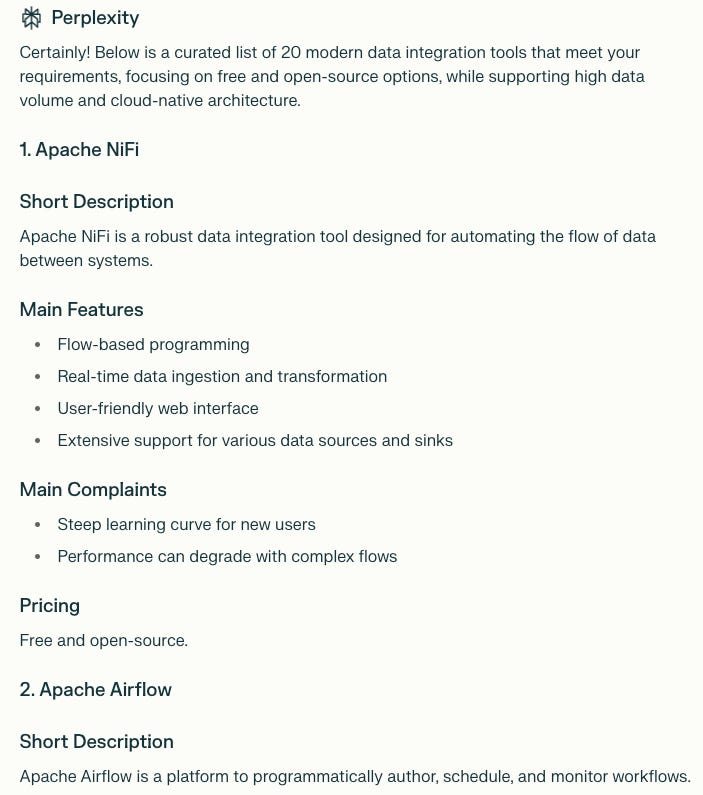
Evaluating Tools Against These Requirements
Once you have your requirements, use a comparison table to evaluate tools based on these criteria. Here's what the AI generated for me:
Real-World Exercise #2: Using the requirements you documented in Exercise #1, create a similar comparison table for 2-3 tools you're considering. Rate each tool based on how well it matches your requirements and identify the top contender.
Tool Selection Checklist
To make tool selection easier, here's a checklist of steps to guide you through the decision-making process:
List your specific requirements (as detailed in Step 1).
Research top tools used in similar projects.
Create a comparison table and score each tool.
Run a small-scale test with your top choice, if possible, using sample data.
Evaluate the test results based on performance, ease of use, and integration.
By following this checklist, you can systematically evaluate tools and select the best fit for your project's needs.
Did you know? I wrote an extensive Snowflake learning guide. And you can have this for free!
You only need to share Data Gibberish with 5 friends or coworkers and ask them to subscribe for free. As a bonus, you will also get 3 months of Data Gibberish Pro membership.
Research Leve #2: Learning from Large-Scale Data Architectures
One of the best ways to advance your skills is to learn from companies that have successfully solved a problem at scale. Observing how companies like Netflix, Airbnb, or Google structure their data architectures can provide insights and inspiration.
How to Study Company Data Infrastructures
Check for companies with similar data challenges. For example, if you’re building a recommendation engine, Netflix and Spotify are relevant; if you’re managing a marketplace, look at Airbnb or eBay.
With a well-crafted prompt, AI can summarise a company’s data infrastructure. You can cover key aspects like data ingestion, storage, processing, and analytics.
For example, you can ask AI to describe Netflix’s real-time data pipeline, Uber’s approach to low-latency processing, or Airbnb’s use of data lakes and meshes. AI will provide you with a concise overview of each architecture’s core components, tools, and design choices.
Real-World Exercise #3: Research how Netflix, Airbnb, or Google structure their data architectures. Summarise the most relevant aspects and consider how to adapt them to your organisation's needs.
Here's the basic sample prompt:
Please explain the data infrastructure at Airbnb.
Keep it high-level and explain the most important details.
And, this is the beginning of the response to the advanced prompt:
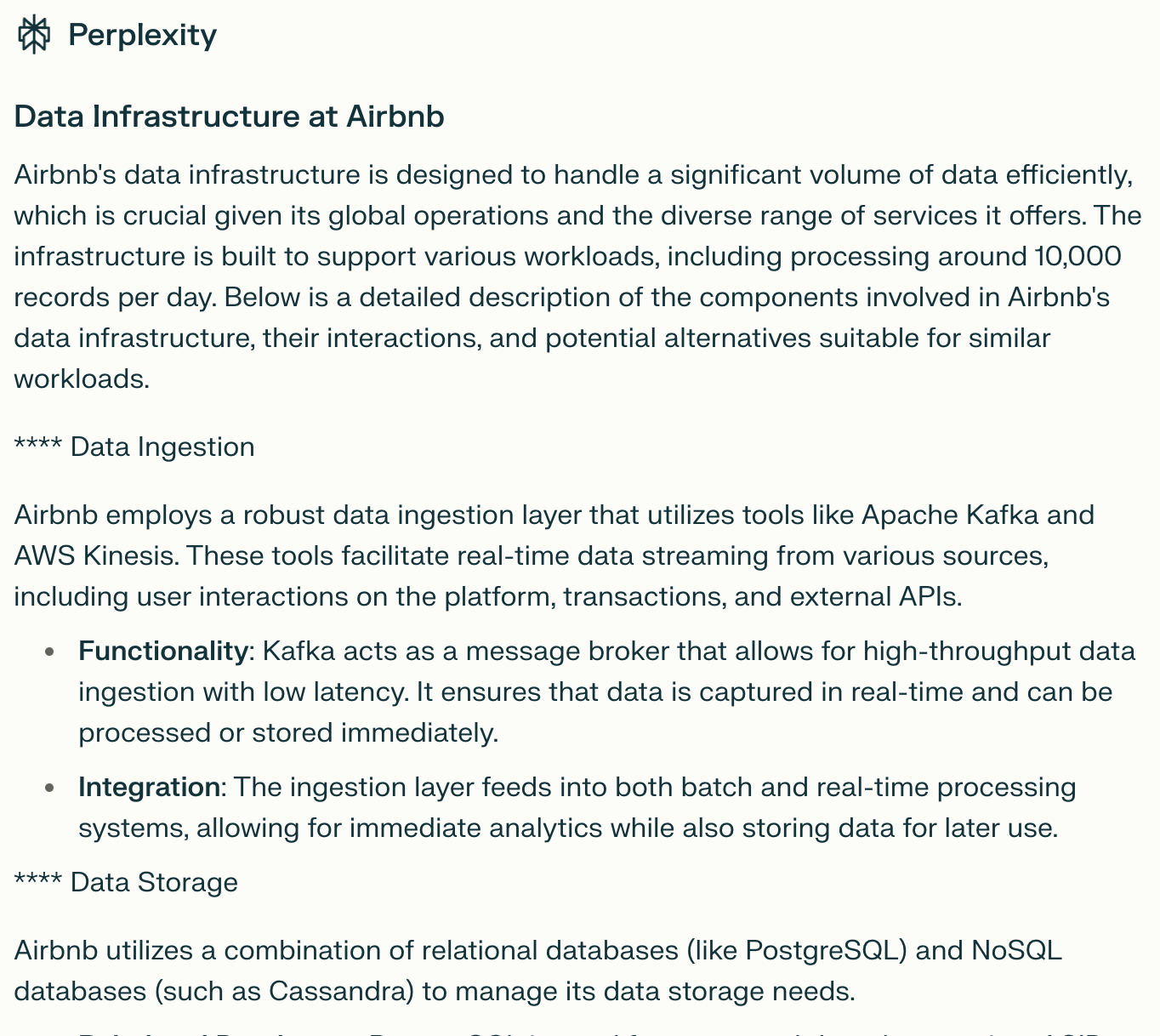
Example Architecture Chart with Mermaid
Reading the text is great, but I need more. I want to see a high-level diagram of the infrastructure I research.
To do that, I use Mermaid charts. So, once you have a good explanation of what you need, you can ask Perplexity to write the Mermaid code for you.
Then, you can generate the image. I usually use their official service to do that.
Real-World Exercise #4: Use your AI generated research. Ask the AI to draw an infrastructure diagram for you.
Here's what the AI generated based on my Airbnb research.
Combining the diagram with the text makes your research 10x faster!
Research Level #3: Using AI to Assess and Refine Your Data Infrastructure
After researching tools and learning from industry examples, the next step is to put these insights into action. AI can help you assess your current infrastructure, identify bottlenecks, and guide the design of a data platform.
Even if you've identified promising tools and architectures, testing these assumptions and getting data-driven insights is critical.
Explain Your Architecture and Requirements to AI for Feedback
Prepare a draft of your architecture and requirements. Then, you can validate your assumptions.
To get the most relevant feedback, provide the AI with a detailed description of your data platform. Here's a checklist of key information to include in your prompt:
Architecture Overview: Describe the high-level structure of your data platform, including key components. Explain how data flows through each stage.
Requirements and Goals: Define your performance, scalability, and cost goals. For example, you might aim to reduce query latency by 20% or handle up to 1 TB of streaming data per hour.
Expected Workloads: Mention the types and volume of data you expect to handle. Include details like batch vs. real-time processing and any peak usage times.
Current Tooling: List the specific tools and services you plan to use or are considering (e.g., Apache Kafka for ingestion, Snowflake for storage, Spark for processing).
Potential Pain Points: Highlight any known challenges or uncertainties. For instance, if you need clarification on scaling limits, mention this so the AI can provide targeted feedback.
Real-World Exercise #5: Describe your architecture and requirements in detail, using the checklist above as a guide. Input this prompt into Perplexity and document the feedback you receive. Review the suggestions and note which ones align with your goals, prioritising them for implementation in the next step.
As before, here's your basic prompt:
We're designing a data platform with an ingestion layer using Kafka, a storage layer with Snowflake, and a processing layer with Spark.
We aim to maintain query latency under 200 ms, handle 1 TB of streaming data per hour, and keep storage costs within $10,000/month.
Are there any potential bottlenecks or scalability issues we should consider? Can you suggest optimisations for this setup?
And always, here's what I get when I run the advanced prompt:
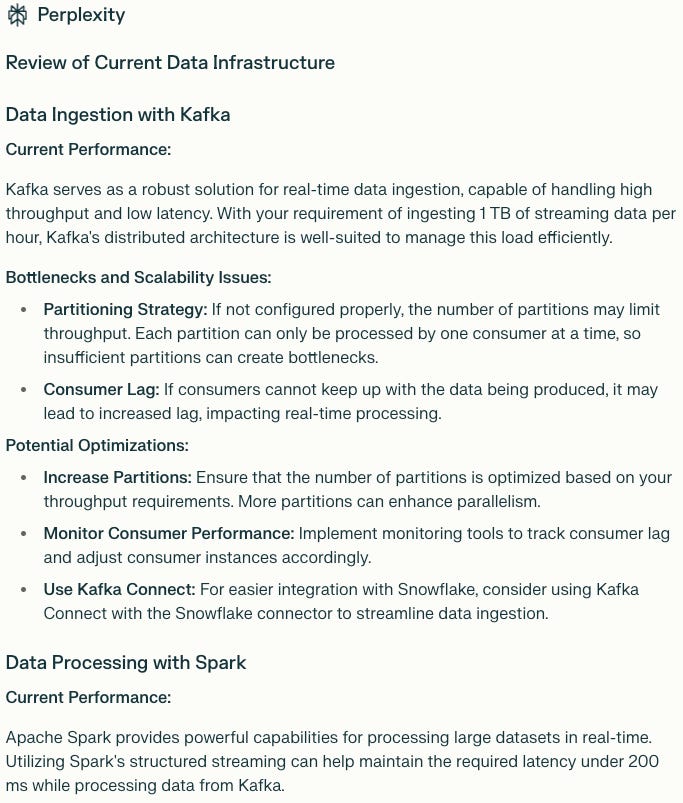
If you have enjoyed the newsletter so far, please show some love on LinkedIn and Threads or forward it to your friends. It really does help!
Iteratively Implement AI-Recommended Improvements
With feedback in hand, you're ready to start implementing changes to improve your infrastructure. Instead of overhauling your entire platform at once, focus on incremental improvements based on AI recommendations. This iterative approach allows you to test each change, measure its impact, and adjust as needed, reducing the risk of disruptions.
Select High-Impact Changes: Start with low-risk, high-impact changes recommended by AI, such as optimising a storage configuration or adjusting query processing settings. Document the specific metrics you expect to improve (e.g., query latency, cost efficiency).
Implement and Test: Make the changes in a controlled environment (e.g., a staging area or sandbox) to assess their impact. Use performance benchmarks and cost tracking to measure results.
Monitor and Adjust: After implementing each change, monitor key metrics over time. AI-powered monitoring can alert you to unexpected changes, such as a spike in latency or resource usage.
Gather Feedback and Iterate: Once you've tested a change, use AI to analyse the results. AI can provide further guidance on adjustments or alternative solutions if the improvement doesn't yield the expected outcomes.
Real-World Exercise #6: Implement one high-impact recommendation from the AI feedback in a test environment. Monitor performance, resource usage, and costs over a designated period. Adjust based on results, and repeat the process with additional improvements as you progress.
Here's a sample improvement cycle using AI feedback to optimise query latency in a data platform:
In this example:
AI Feedback identifies in-memory caching as a potential solution to improve query latency.
Implementing the change in a test environment allows you to gauge its impact without risking production stability.
Monitoring ensures that you catch any unintended side effects (e.g., excessive memory usage).
If the change doesn't yield the desired results, you can seek further AI feedback to adjust the approach.
Final Thoughts
Most people use AI for boring tasks. But AI can be a real competitive advantage for data engineers.
Research is often tedious and not very rewarding. But innovation and optimisation are great. Often, you wish somebody could tell you the answer right away.
Traditionally, only consultants and senior data engineers have the opportunity to work on these challenging and rewarding projects. Nowadays, everybody can research and validate ideas.
Now, here's the problem: You can't just rely on the AI. Remember, AI doesn't innovate. It only reproduces what others have done. At this stage, only people can invent groundbreaking solutions.
I don't know if this is good or bad for the industry. But I know that if you don't use AI today, you are on your way to extinction like a dinosaur.
AI doesn't make the job market tougher. It doesn't remove people from the workforce. It changes the rules. It redefines responsibilities. It's up to you if you extinct or thrive as a data engineer.
I believe that weekly newsletters are insufficient. A group of leaders and I launched an exclusive Discord community where you can dive into vibrant discussions on software and data engineering, leadership, and the creator economy.
Join today, and let's supercharge our professional journeys together!
Summary
AI is everywhere, but most resources only scratch the surface. As a data engineer, you need more than basic tutorials. You need practical, actionable insights for building a scalable, high-performance data platform.
In this article, you learned three best practices for putting AI to work in data engineering:
Researching and Selecting Tools: Define your project requirements, shortlist tools, and use AI to create a data-backed comparison. This ensures you pick the right tools for the job without guesswork.
Learning from Large-Scale Architectures: Study how companies like Netflix and Airbnb design their data systems. By adapting proven architectures, you can structure a more resilient platform. Use AI to summarise and visualise these designs so you save time.
Assessing and Refining Your Infrastructure with AI: Feed your architecture into AI for feedback on bottlenecks, scalability, and costs. Then, make incremental improvements, test them, and repeat. This iterative process lets you fine-tune your platform with data-driven precision.
Today, AI lets you access insights that were once reserved for consultants and top engineers. But remember: AI can't innovate; it only amplifies existing knowledge. It's up to you to push boundaries and build a platform that meets your goals.
If you're not using AI yet, you're missing out. AI won't replace you, but it's changing the rules. The choice is simple—adapt and thrive or risk being left behind.
Until next time,
Yordan
How Am I Doing?
I love hearing you. How am I doing with Data Gibberish? Is there anything you’d like to see more or less? Which aspects of the newsletter do you enjoy the most?
Use the links below, or even better, hit reply and say “Hello”. Be honest!